Along with training, AI inference is one of the primary functions that generative artificial intelligence models perform.
Large language models like ChatGPT and image generator tools work by making inferences from user prompts, based on information they already have.
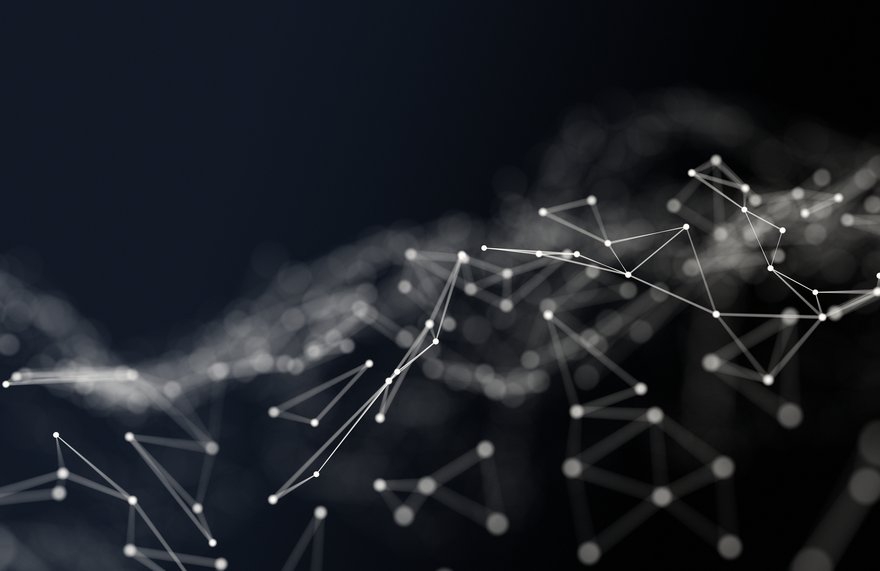
Definition
What is AI inference, exactly?
AI inference describes the process of a machine learning model making deductions based on information it's been trained on.
Inference follows the training stage to make an AI model work. It happens when you give an AI model like ChatGPT a prompt and get a response. The response is based both on the data that the AI model has been trained on, and on its ability to infer answers.
Why is it important?
Why is AI inference important?
The two components that differentiate AI models from one another are training and inference. The two work closely together. Training, consisting of a set of billions or even trillions of parameters, directly influences a model's ability to infer. The better trained it is, the better its inferences will be.
Training is key in determining a model's strength, but inference ultimately determines its accuracy. Although the strength of the training dataset influences inference, a model is not worth much without the model to infer.
Inference enables self-driving cars to drive safely on roads they've never been on before, and allows large language models to answer questions they haven't seen before. Without inference, AI models wouldn't be able to do new things.
Most of an AI model's life is spent in inferencing since training takes place before a model is ready to be used.
How to evaluate
How to evaluate AI inference
Running AI models is typically expensive, as it requires a lot of power to perform the millions of computations necessary for large language models like ChatGPT to work.
As much as 90% of a model's time can be spent on inference, which continues after the model is deployed; training is a one-time cost. So, in active models, training tends to be less important than inference.
There are several ways to evaluate the inferential abilities of large language models. Speed is a key differentiator between models, and models with better inferencing capabilities will result in faster response times.
Cost is another area worth evaluating, especially if you're paying to run the model, and there are tools and techniques that can help reduce the cost of running expensive AI models. Arm's (ARM -3.43%) CPU architecture, used in almost every smartphone, is being adopted for AI because of its efficiency.
Accuracy is also a valuable way of judging inference capabilities, and inference and training both determine the accuracy of a model. Accuracy can be easily tested by posing the same questions to different models and seeing which ones give the best answers and make the fewest mistakes.
Related investing topics
How AI inference works
How it works
One example of AI inference is a self-driving car that can recognize signage on the road -- even if it hasn't been on that road.
Inference also gives AI predictive abilities, so it can predict what you're about to type or make forecasts from a given set of information.
As inference enables humans to make decisions and judgments, inference does the same thing for artificial intelligence.
As models get more powerful, the inference is likely to become even better, making progress toward artificial general intelligence (AGI). AGI will only be reached when inference capabilities that can match those of humans.