David Gardner wrote on our discussion boards, "Investing in Amazon -- like investing in any Rule Breaker -- is an act of faith: a belief in things unseen." Among the controversial remarks David has recorded on the Fool, perhaps this one has drawn the most attention.
I understand most of the criticism from fellow Fools. In a world of technological breakthroughs, appealing to faith is not something people do lightly these days -- not in public anyway. The topic is approached most delicately, lest one be lumped with astrologers on late-night infomercials. If we seek to educate beginning investors, we have an obligation to be as deliberate and as quantitative as possible in our approach. Right?
Not necessarily.
Yet, it's really odd that I find myself saying this, considering my background. I devoted 10 years of my life to getting a master's degree in statistics and learning to apply these quantitative tools to optimizing manufacturing processes. Ironically, it's precisely this extensive experience with the language of science that led me to consider David's non-scientific approach thoughtfully when I first came across it, while still employed in corporate manufacturing. Ten years earlier, I would have summarily dismissed the Rule Breaker strategy without much thought. Soft nonsense, I would have muttered.
But, the deeper I got into applying statistics to manufacturing, the more often I bumped into that hard-to-pin-down, but ultimately very real, boundary between knowledge and belief. And, I was bumping into it far earlier than I ever believed I would. Not only that, as I became more skilled in applying my advanced quantitative skills, I still could not touch the business contributions of my coworkers who operated less strictly on the knowledge side of the divide.
An example of how statistics can fail
Let's say that you are working on a long, complex manufacturing process with many sequential modules. One of your engineers comes up with a new design for one of these modules that is supposed to improve the reliability of the overall process by reducing the rate of "process upsets." These process upsets generate poor-quality product and costly machine downtime.
But, since the new module design is significantly more expensive, your job is to evaluate its performance first, and decide if the improvement claim can be confirmed before the money is allocated. In other words, no one wants to pay for this thing on faith. They want facts -- statistical facts.
Easy, right? Just take some baseline data, install the new module, and take some more data. If you are really ambitious, take a little more data after reinstalling the original module. Then carry out those fancy statistical tests that are now second nature and draw a conclusion.
In practice, it never worked out this way. Time after agonizing time, it turned out to be much more complicated than we expected and, almost without fail, our final decision was as much based on faith as on data. Of course, we didn't use the word faith. We preferred the term technical judgment. But, the bottom line is that we rarely proved anything to any convincing degree.
Lost in numbers, without resolution
We didn't prove anything statistically, even though I can manipulate numbers with the best of them, so that wasn't the issue. The trick was always in generating the numbers. I remember creating a remarkably complex model for a particularly impenetrable data set. After allowing me to struggle with it for a few days -- as a learning experience -- my esteemed mentor stopped by my office and casually remarked: "You know, you can create the most monumental statistical edifice possible, but it still won't hide the fact that you don't have a lot of good information here."
The problem in collecting good data was always the same. Once you get it, it's painfully simple, but the bottom line is that hardly anybody gets it. For the longest time, I didn't either. The problem is always capturing all the relevant sources of variability. In order to get meaningful data, you have to either control or randomize across every one of them.
For example, is it enough just to try the prototype model? How do we know it isn't better or worse by virtue of the quality of its workmanship than the later production models? To eliminate this frequently important variable, one really ought to trial two or three of each module type. Imagine trying to convince the guys on the floor that the hours of down time and lost production required to carry this out will be worth it. Not a good way to make friends.
And, this is just the tip of the iceberg. You have to control for raw material variability, sampling finished product, controlling testing conditions, and so on. As the years went on, I got deeper and deeper into the quantitative, technical hole, trying to solve these problems. I gained the respect of my peers for my technical skills, but it became clear to me that I was not able to turn these skills -- as hard as they were to explain to the untrained -- into clear results for the business. Meanwhile, all around me it was very clear that others were moving the business forward in very tangible ways -- and many of these folks had not even mastered simple spreadsheets.
Investing, statistics, and faith
Now, compare this scenario with evaluating investing strategies. How would I go about proving that a particular strategy -- say the Rule Breaker Portfolio -- is effective? Well, to start with, all the sources of variability have to be controlled. This means that, at a bare minimum, multiple people should employ the strategy in multiple markets (like multiple modules across different raw materials). And, how do we sample markets of the future? Markets evolve. They don't necessarily repeat.
At its core, the scientific method requires the ability to replicate results across like situations. But, coming up with "like situations" in investing makes industrial experiments look like bubble-gum-card trading. Take it as fact -- no investing strategy will ever be proven. None will be a lock. Don't be taken by impressive quantitative strategies. Complexity is never a guarantee of anything but complexity.
So, what's the point? Am I suggesting that we all model our investment strategies after the infamous dart-throwing monkeys? Nope. Not at all.
To the extent we do decide to pick individual stocks, though, I am saying that we shouldn't be afraid to add faith to our investing toolkit. There is nothing embarrassing about it. By all means, study your investments in detail, particularly if you enjoy the process. You'll learn valuable lessons for life and for your own business career, even if you don't come out ahead of the market.
Ultimately, though, being human is all about not knowing what happens next, and all investing comes down to belief. Whether it's medicine, manufacturing, microchips, or investing, there will always be a point at which knowledge ends and faith begins. And, in investing, most people get to this point much sooner than they want to admit.
To close tonight, if you care to take a break from financial statements to read a very thoughtful essay, I highly recommend "Eyes Wide Open," a New York Times Magazine article (free registration) by Richard Powers. I stumbled across this essay as it sat printed, but forgotten, on the office printer. (How's that for fate? I later learned it was co-Rule Breaker Jeff Fischer who printed it.) The article approaches the line between faith and knowledge from the opposite direction, starting in history and moving forward.
--Buster
You're reading a free article with opinions that may differ from The Motley Fool's Premium Investing Services. Become a Motley Fool member today to get instant access to our top analyst recommendations, in-depth research, investing resources, and more. Learn More
Investing on Faith
A former statistician explains why all the number-crunching in the world is no substitute for faith in your investing strategy.
Invest Smarter with The Motley Fool
Join Over Half a Million Premium Members Receiving…
- New Stock Picks Each Month
- Detailed Analysis of Companies
- Model Portfolios
- Live Streaming During Market Hours
- And Much More
Motley Fool Investing Philosophy
- #1 Buy 25+ Companies
- #2 Hold Stocks for 5+ Years
- #3 Add New Savings Regularly
- #4 Hold Through Market Volatility
- #5 Let Winners Run
- #6 Target Long-Term Returns
Why do we invest this way? Learn More
Related Articles





Motley Fool Returns
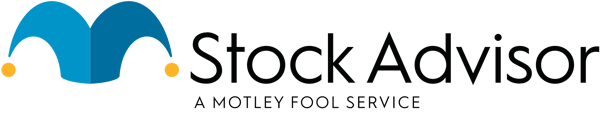
Market-beating stocks from our award-winning analyst team.
Calculated by average return of all stock recommendations since inception of the Stock Advisor service in February of 2002. Returns as of 05/10/2024.
Discounted offers are only available to new members. Stock Advisor list price is $199 per year.
Calculated by Time-Weighted Return since 2002. Volatility profiles based on trailing-three-year calculations of the standard deviation of service investment returns.
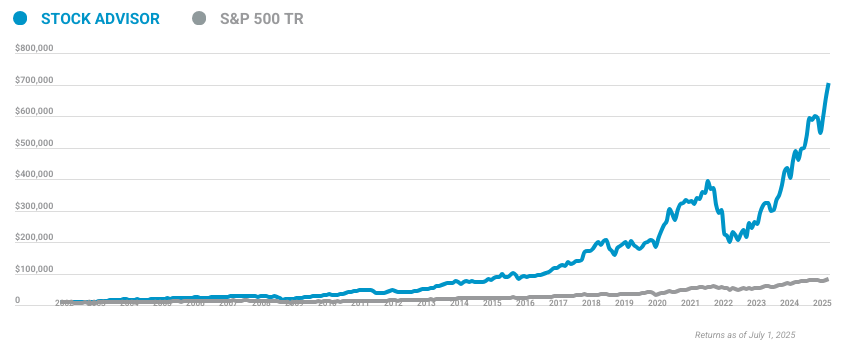
Premium Investing Services
Invest better with The Motley Fool. Get stock recommendations, portfolio guidance, and more from The Motley Fool's premium services.