From intelligently curated web search results to self-driving cars and smart speakers, artificial intelligence (AI) is already playing an important role in our lives. But this is just the beginning given the convenience that this technology brings.
This is why several companies across different industries are looking to embrace AI as fast as possible, giving investors a ton of options to buy into AI's growth. But one of the best ways for investors to take advantage of this fast-growing tech trend is by investing in NVIDIA (NVDA 2.53%), which is enabling AI technology with its graphics cards.
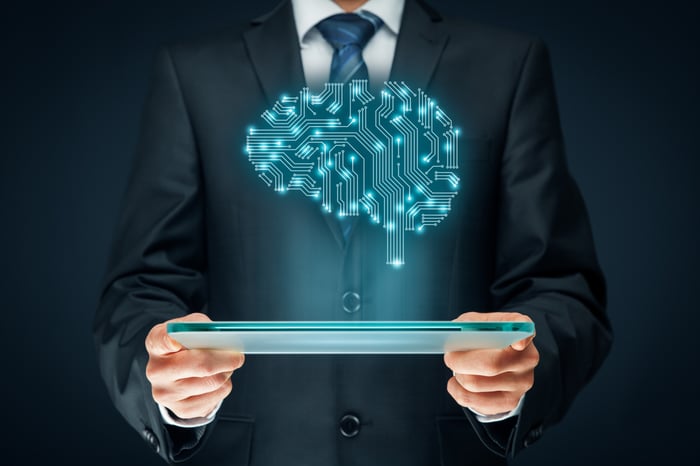
Image Source: Getty Images.
NVIDIA GPUs are critical for AI's growth
NVIDIA is considered to be one of the best AI bets out there, and for good reason. Its graphics processing units (GPUs) are critical for the training of AI models by processing large data sets in a short time while keeping infrastructure costs and power consumption low. GPUs have hundreds of cores and thousands of threads that allow them to rapidly carry out complex calculations.
Back in 2012, Google created a network of 1,000 CPUs and trained the same to spot cats in YouTube videos. This system cost the search engine giant $1 million at that time. But the same system can now be built at a fraction of the cost thanks to the superior tech specs of GPUs. That's because 1,000 CPUs have a total of 16,000 processing cores, while just three GPUs can do the job since they have a combined core count of 18,000.
In fact, Stanford researchers built a slightly better system using GPUs and spent just $33,000. NVIDIA claims that its Tesla V100 GPU accelerator can perform the task of 100 CPUs at once as it has more than 5,000 cores. For comparison, Intel's top-of-the-line seventh-generation Core i9-7980XE processor has 18 cores and is priced at nearly $2,000, while the NVIDIA Tesla V100 goes for approximately $11,000.
This massive advantage that GPUs enjoy over CPUs makes them ideal for deployment in data centers where AI engines are trained. Not surprisingly, NVIDIA's data center business is witnessing massive growth thanks to the booming demand for its Tesla GPUs, as major cloud players are deploying its chips.
Microsoft recently added support for the NVIDIA GPU Cloud to its Azure cloud platform for the training and inferencing of AI models. Other key cloud players such as Amazon and Baidu are already accelerating their data centers with the help of Tesla GPUs.
The graphics specialist's data center sales jumped 82% annually last quarter to $760 million, accounting for just over 24% of total revenue. By comparison, this segment was contributing around 12% to NVIDIA's total business just two years ago.
Moving to the next phase
The good news is that NVIDIA is now looking to push the envelope further. The company recently unveiled the Tesla T4 data center GPU for inferencing purposes based on the latest Turing architecture, which should allow it to tap into yet another lucrative niche.
So far, GPUs have been deployed extensively for training purposes when a ton of data needs to be processed to help the model learn, but inferencing is a different ball game, as the model has already been trained and optimized by that time.
Data centers need fewer resources in the inferencing phase as compared to training, and there are other chips that can do the job in a more efficient way. Intel and Xilinx, for instance, are looking to push their programmable chips into data centers for inferencing purposes, and they have found some success on this front.
Microsoft decided to offer Intel FPGAs (field-programmable gate arrays) earlier this year for real-time AI inferencing, while Amazon is offering Xilinx's chips for machine learning inference and other applications. The advantage of FPGAs over GPUs is that they deliver a better performance per watt of power consumed, and they can be optimized to perform specific tasks thanks to their flexible architecture, something that's not possible with GPUs that are based on a fixed architecture.
As such, FPGAs are suited for large-scale deployment in data centers for inferencing to keep energy consumption low and deliver an efficient performance. But NVIDIA doesn't want to lose out on the inferencing market, which it believes will be worth $20 billion in the next five years.
This is why the company has launched a GPU purpose-built for real-time inferencing and fit for deployment in large-scale servers thanks to its power-efficient nature. What's more, NVIDIA boasts that the T4 can perform up to 40 times faster than a CPU during inferencing, and it has already scored a customer: Google.
The Alphabet subsidiary will soon start supporting the Tesla T4 GPUs on the Google Cloud Platform, and this will be just the beginning. Server original equipment manufacturers such as Dell EMC, IBM, Fujitsu, Hewlett-Packard Enterprise, and Cisco are planning to offer servers based on the Tesla T4.
NVIDIA is just getting started
Demand for artificial intelligence chips is going to expand exponentially in the future. Allied Market Research estimates that the global AI chip market will be worth more than $91 billion in 2025 as compared with just $4.5 billion last year. Additionally, the chipmaker is targeting other fast-growing AI applications such as autonomous cars, paving the way for GPU sales beyond the data center.
This makes NVIDIA a solid play on the growing demand for AI chips, as it not only supplies the basic building blocks to enable this technology, but also dominates the GPU market.