Artificial intelligence (AI) is an area that focuses on enabling machines and software to process information and make decisions autonomously. Machine learning, a component of AI, involves computer systems enhancing their problem-solving and comprehension of complex issues through automated techniques.
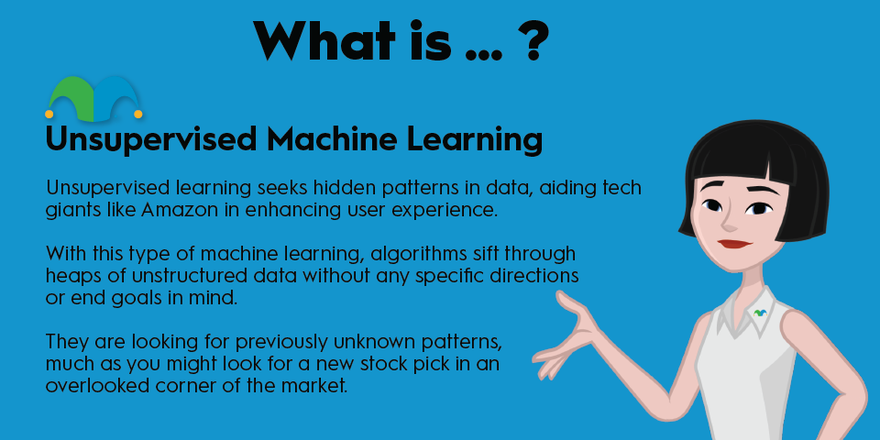
The three central machine-learning methodologies that programmers can use are supervised learning, unsupervised learning, and reinforcement learning. For in-depth information on supervised machine learning and reinforcement machine learning, kindly refer to the articles dedicated to them. Here you can read up on the basics of unsupervised machine learning.
What is it?
What is unsupervised machine learning?
With unsupervised machine learning, a system is like a curious toddler exploring a world they know nothing about. The system is exploring data without knowing what it's looking for but is excited -- in a digital kind of way -- about any new pattern it stumbles upon.
With this type of machine learning, algorithms sift through heaps of unstructured data without any specific directions or end goals in mind. They are looking for previously unknown patterns, much as you might look for a new stock pick in an overlooked corner of the market. This is rarely the last step since the owner of the raw data typically applies more sophisticated deep learning or supervised machine learning analyses to any potentially interesting patterns.
Implications and intricacies
The implications and intricacies of unsupervised learning
Why should you care about this artificial intelligence toddler on a quest without a firm goal? Well, unsupervised machine learning is actually on the cutting edge of technology and innovation. It’s a key player in everything from autonomous vehicles learning to navigate roads to recommendation algorithms on your favorite streaming platforms. This pattern-finding method is a powerful first step in a deep analysis of any complex topic, from weather forecasting to genetic research.
Two major types of unsupervised learning are clustering and association.
- Clustering is like sorting a pile of random stocks into sectors with some common theme or quality. It's all about grouping similar things together.
- Association, on the other hand, is like realizing that smartphone component stocks often rise when Apple (AAPL -0.35%) announces a new iPhone. It’s about finding relationships and connections between apparently separate things.
How it's used
Making good use of unsupervised machine learning
So now you know what unsupervised machine learning is and why it matters. How can you take this newfound knowledge and put it to good use?
First off, you can make informed investment decisions. Companies leveraging unsupervised learning are often poised for growth as this technology continues to evolve. Think about Amazon (AMZN 3.43%) using unsupervised learning for its product recommendations or Netflix (NFLX -0.63%) running unsupervised machine learning routines across years of collected viewership data to generate your streaming home page and make future content production decisions.
These applications aren't just fun toys -- they are business advantages and growth drivers.
Also, AI and machine learning continue to reshape many industries. Whether you're into FAANG stocks or emerging AI startups, knowledge about unsupervised learning can give you an edge in evaluating a company's tech prowess and potential for future success.
Related investing topics
Social media connections
Unsupervised learning in action: Social media connections
We all appreciate a bit of connection, right? Well, thanks to unsupervised machine learning, we're getting better at finding people we might know or like on social media platforms. Facebook is a prime example.
Have you ever wondered how Facebook seems to know who your actual friends from high school are -- the ones you may actually want to keep in touch with? It's not sorcery. It's unsupervised learning in action.
Meta Platforms' (META 0.43%) massive social network continually analyzes a trove of user data, looking for patterns and shared features among users. Common friends are a helpful clue; similar locations and shared interests can point the platform in the right direction, and mutual workplaces can be the clincher. None of these qualities is enough in itself to find that long-lost flame or forgotten friend, but they add up through the power of unsupervised machine-learning algorithms.
So when Facebook suggests "People You May Know," it essentially gives you the output of an unsupervised learning model. The social network isn't just pulling these suggestions out of a digital hat. Each one is the result of a complex analysis of patterns and connections.